COLLOQUIUM
Pooyan Jamshidi
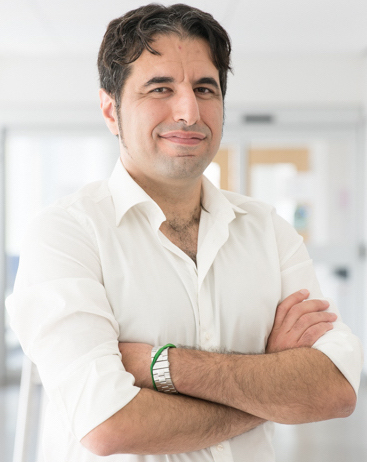
Abstract
A wide range of modern software-intensive systems (e.g., autonomous systems, big data analytics, robotics, deep neural architectures) are built configurable. These systems offer a rich space for adaptation to different domains and tasks. Developers and users often need to reason about the performance of such systems, making tradeoffs to change specific quality attributes or detecting performance anomalies. For instance, developers of image recognition mobile apps are not only interested in learning which deep neural architectures are accurate enough to classify their images correctly, but also which architectures consume the least power on the mobile devices on which they are deployed. Recent research has focused on models built from performance measurements obtained by instrumenting the system. However, the fundamental problem is that the learning techniques for building a reliable performance model do not scale well, simply because the configuration space is exponentially large that is impossible to exhaustively explore. For example, it will take over 60 years to explore the whole configuration space of a system with 25 binary options.
In this talk, I will start motivating the configuration space explosion problem based on my previous experience with large-scale big data systems in industry. I will then present my transfer learning solution to tackle the scalability challenge: instead of taking the measurements from the real system, we learn the performance model using samples from cheap sources, such as simulators that approximate the performance of the real system, with a fair fidelity and at a low cost. Results show that despite the high cost of measurement on the real system, learning performance models can become surprisingly cheap as long as certain properties are reused across environments. In the second half of the talk, I will present empirical evidence, which lays a foundation for a theory explaining why and when transfer learning works by showing the similarities of performance behavior across environments. I will present observations of environmental changes‘ impacts (such as changes to hardware, workload, and software versions) for a selected set of configurable systems from different domains to identify the key elements that can be exploited for transfer learning. These observations demonstrate a promising path for building efficient, reliable, and dependable software systems. Finally, I will share my research vision for the next five years and outline my immediate plans to further explore the opportunities of transfer learning.
Pooyan Jamshidi is a postdoctoral researcher at Carnegie Mellon University, where he works on transfer learning for building performance models to enable dynamic adaptation of mobile robotics software as a part of BRASS, a DARPA sponsored project. Prior to his current position, he was a research associate at Imperial College London, where he worked on Bayesian optimization for automated performance tuning of big data systems. He holds a Ph.D. from Dublin City University, where he worked on self-learning Fuzzy control for auto-scaling in the cloud. He has spent 7 years in industry as a developer and a software architect. His research interests are at the intersection of software engineering, systems, and machine learning, and his focus lies predominantly in the areas of highly-configurable and self-adaptive systems (more details:
https://pooyanjamshidi.github.io/research/).
Date: Feb. 26, 2018
Time: 10:15-11:15 am
Place: Innovation Center, Room 2277